John Cvetko
TEK Associates (USA)
Tamper Detection in AI Systems: A Framework for Safety and Robustness in LLMs
Large Language Models (LLMs) have transformed natural language processing with their ability to generate human-like text, but their susceptibility to tampering poses significant challenges to their reliability and trustworthiness. Malicious actors can exploit these vulnerabilities through methods like data poisoning, backdoor implantation, or bias injection, resulting in compromised outputs that can propagate disinformation or distort decision-making processes. This paper introduces a novel evaluation framework designed to systematically detect and analyze potential tampering throughout the LLM lifecycle. By integrating multi-dimensional metrics across robustness, bias detection, and security, the framework provides a comprehensive approach to safeguarding LLMs. Drawing on advancements in adversarial testing, bias attribution, and data forensics, the framework bridges theoretical insights with practical methodologies to ensure the secure and fair deployment of LLMs in critical applications.
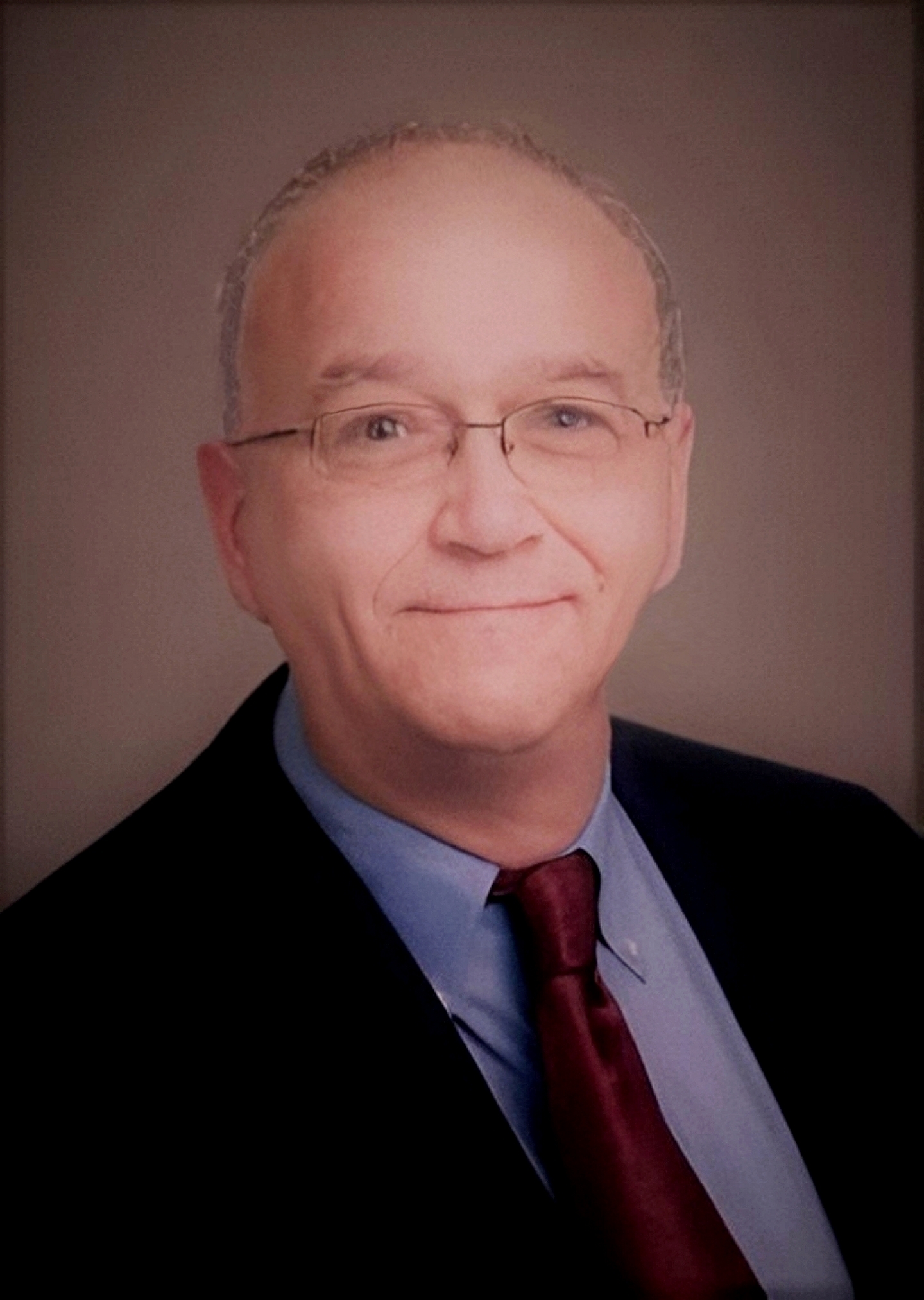